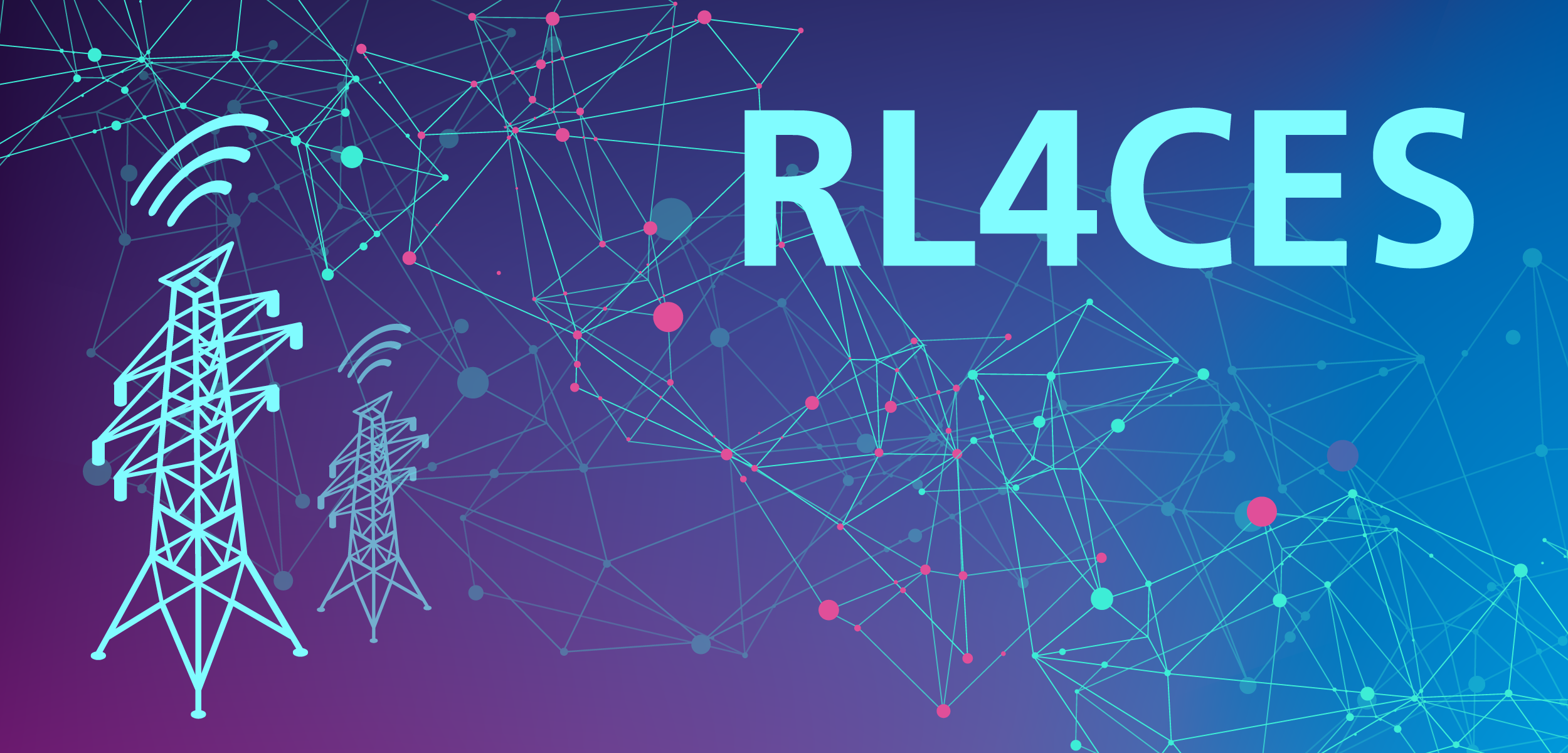
Reinforcement Learning for Cognitive Energy Systems
Advancing Reinforcement Learning in the Energy Industry
We, the junior research group, RL4CES - Reinforcement Learning for Cognitive Energy Systems,aim to realize the potential of Deep Reinforcement Learning (Link DRL) in the context of the energy system.
With our Research we seek to make Deep Reinforcement Learning safer, more effective and more cost-effective for the energy industry and thus make a significant contribution to a stable and resilient energy system.
The focus of our application is on the topics of automated grid control and automated energy trading.
News-Feed
- Workshop: Machine Learning for Sustainable Power SystemsDer Workshop Machine Learning for Sustainable Power Systems (ML4SPS) bringt WissenschaftlerInnen aus den Feldern Machine Learning und Energiesysteme auf der European Conference of Machine Learning zusammen. Forschende und AnwenderInnen können ihr Wissen und ihre Erfahrungen in den Feldern erneuerbare Energiesysteme, Grid Management und Machine Learning teilen und so von der Expertise der Community profitieren. Zwei… Workshop: Machine Learning for Sustainable Power Systems weiterlesen